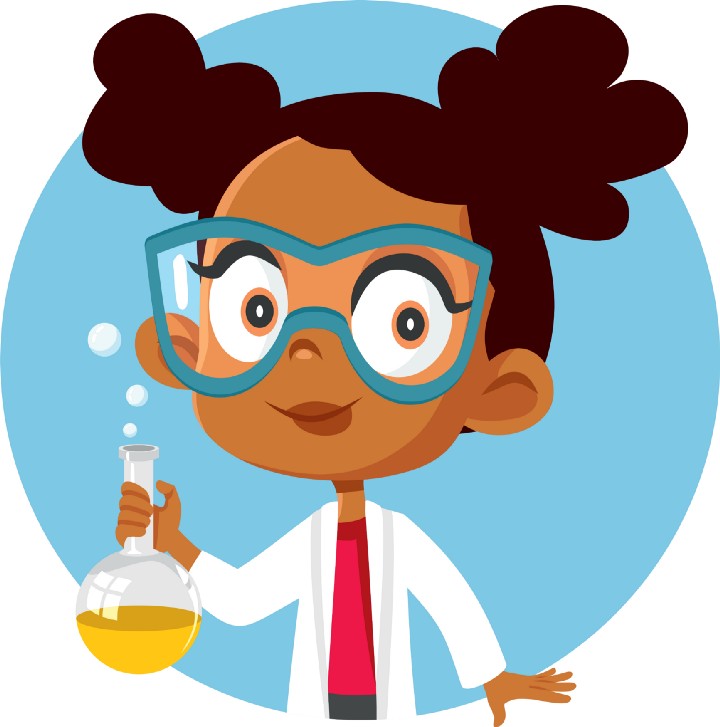
Remember when ads worked just on a “spray-and-pray” strategy? The common refrain of marketers was that “half of my ad spend works well, I just don’t know which half.”
How that world has changed. The world of digital marketing has almost entirely been made a science by the likes of Google and Facebook. And the key behind all of this has been user targeting. And now, with sophisticated AI models like the ones we use at StepFunction, we are able to do the same for SaaS Ops processes like customer retention and upsell. The key is to know how to leverage data to begin turning what used to be an opaque art into hard science.
A large SaaS enterprise approached us last year frustrated that their customer success efforts weren’t resulting in meaningful impact. It wasn’t for lack of effort — they had put in place good processes and strong teams that worked to engage customers at every opportunity. And yes, some metrics like NPS scores and even churn rate showed positive movement. But the results were underwhelming when the impact was looked at in terms of dollars retained and grown. Their Net Revenue Retention rate (NRR) remained anemic.
We quickly saw that they had streamlined their processes but hadn’t employed the science of customer targeting, which would substantially boost NRR. Were they really trying to save, renew or upsell to the optimal set of customers? For example, in their churn save efforts, were these SaaS operators targeting customers who were (a) exhibiting a critical level of dissatisfaction (b) savable, and (c) worth saving?
That’s where StepFunction’s Customer Intent Engine™ (CIE) comes in. We ingest an extraordinary range of customer behavior signals — product usage, support tickets, survey feedback, CRM, and social media signals to name a few — over a long period of time. We then deduce end customers’ intent, infer the associated risks or opportunities, and go about increasing NRR one scientifically-optimized customer at a time.
Let’s take a look at the different key categories below.
A subscriber churns when they terminate their relationship with the SaaS provider. This can happen at any time for month-to-month customers or at renewal time for customers under contract.
How do you determine that a customer is at risk of churn? Sometimes the red flags are clear. For example, certain calls into the company’s call center or support tickets can display clear dissatisfaction. Or product usage can suddenly fall off a cliff. The problem is that these are most often lagging indicators, so that a response to these signals is a case of too little, too late.
So, how can one be proactive? Many SaaS operators use heuristics such as “whenever product usage falls below a certain threshold”, or “support ticket activity goes above a certain threshold”, they mark that customer as risky. The problem with these heuristics is that they rarely achieve even a decent level of accuracy. There are two main reasons for this: 1. A human presumes a universal relationship between a signal and an intent that may only occasionally be valid, and 2. Heuristics cannot catch complex correlations between a large number of behavior signals (product usage, support tickets, feedback, billing, and payments, etc.) over a long period of time.
The ML models behind StepFunction’s CIE are carefully designed to capture the most subtle correlations between a large number of customer behavior signals and typically take as input more than 100 attributes. Moreover, they are built to learn patterns over a long “time series of data,” e.g. how many logins a user has had each week over the past 2 years. Across all 100 attributes.
A subscriber contracts or downgrades when they transition to a plan of lower dollar value. This can happen either when the subscriber (a) uses fewer of your products or features, e.g. switching to the Basic plan instead of Premium, or (b) reduces the number of users of licenses.
This situation is similar to the previous Churn problem. However, there are important differences that need to be taken into account. For example, if a subscriber company has 10 user licenses, 3 might show alarmingly low usage while the other 7 might be fine and dandy. This looks like they simply didn’t need all of their licenses and should be flagged as a downgrade instead of a full churn risk. The good news is that humans don’t need to intervene and flag such risks (it would be impractical to do so at a large scale) as long as the AI models have been trained correctly. This usually comes down to formulating the problem the right way and providing accurate historical “labels” to the AI, e.g. this customer reduced the number of licenses vs. this customer fully churned.
A SaaS company upsells to a subscriber when they either (a) sell more licenses of the product or feature bundle that the subscriber has been using all along, or (b) upgrade them to a more costly version of the product, e.g. to the Premium plan instead of the Basic one.
This problem of inferring an upsell opportunity is not merely the opposite of inferring a churn or downgrade risk. Yes, you want to target loyal customers for upsell, but there’s more to it than that. Ultimately, the upsell opportunity comes down to the kind of “collaborative filtering” technique that Amazon uses to recommend products to its customers. Generally speaking: “People who are of a certain demographic or exhibit a certain kind of behavior bought this product.”
Let’s take a concrete example. Our client mentioned above is a Communications SaaS provider and sells phone and video conferencing services. They wanted to determine which customers would be most likely to purchase a premium feature bundle with call & conference recording. A well-trained AI will not only be able to pinpoint loyal customers, but also the subset relevant in this case, e.g. law firms that demonstrate a significant need for call recording.
Suppose a month-to-month subscriber of your SaaS product tells you on June 1 that they want out of their contract at the end of the month. Or that they want to downgrade their feature bundle at the end of the month. Or suppose an annual subscriber whose contract is coming up for renewal at the end of June tells you on June 1 that they won’t be renewing.
On the surface, it seems like June 1 is a key date. A number of churn prediction models use that as the “churn date”. However, more often than not, June 1 was merely the date that the subscriber “communicated” their intent to churn and that they made up their mind much earlier. StepFunction is constantly striving to determine when the subscriber made up their mind (which could have been several months earlier) and to uncover the causes of that decision.
As discussed in our previous article Why saving your most at-risk SaaS customers is NOT the best goal, just because you know your riskiest customers doesn’t mean that they are the optimal ones to target during your save efforts. Even if you’ve taken the steps mentioned above to get to more accurate risk predictions, you want to qualify your customers further before they become part of your target hotlist. Our previous article walks you through the considerations (e.g. is the customer savable, worth saving, or too costly to save) that help you prepare the optimal hotlist.
It’s not enough to have a great process in place in order to solve today’s complex business optimization problems, whether it is optimizing online ad placements or maximizing the net revenue retained from an existing customer base. Once StepFunction helped our client above with a refined, optimized hotlist of customers to target, the same amount of effort from the Customer Success team led to much greater savings in retained revenue. Although it started with targeting customers at risk of churn, we soon expanded it to include contraction risk and upsell opportunities. Moreover, CSM’s were able to handle these new efforts easily with the same team size because of all the efficiencies gained by the bullseye targeting inherent in the StepFunction hotlist. The resulting cost savings coupled with the increase in revenue from retained as well as upsold customers amounted to an ROI of more than 10 times the cost of the entire StepFunction-led CSM effort. Oh, the wonders of inserting science into a streamlined process!