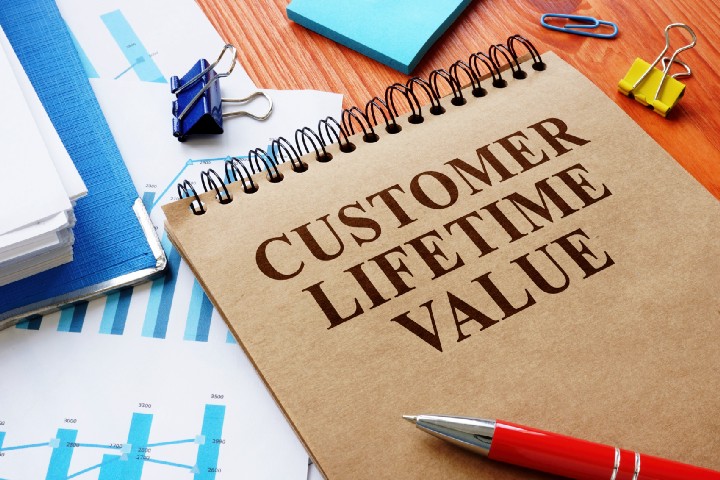
The head of Customer Success at a large organization (90,000 SMB customers) confided to us that she wasn’t sure about a customer retention program they had recently launched. Her lieutenants had carefully designed the program to segment at-risk customers by revenue and offer varying incentives. The program had meaningfully increased retention without inordinate expense. So it was successful — or was it? She wasn’t quite sure if it cost too much for the incremental gain in retention that it brought about. Or maybe the program didn’t go far enough? Increasing the size of the offers could perhaps save more customers and their revenues over time could outweigh the costs. If only she could put a value on saved customers with confidence.
This client isn’t alone. Customer lifetime value (CLV, or, more commonly, LTV) is a net present value estimation of future gains from the customer, but, as physicist Niels Bohr once said, predictions are hard, especially about the future. Also, should we be looking at LTV by individual customer or by cohort of customers?
An estimation of LTV often justifies how much we spend on customer acquisition. LTV and customer acquisition cost (CAC) factor into a very popular metric known as the LTV-to-CAC ratio, and different industry sectors have different benchmarks for this ratio.
Suppose you have a portfolio of 100,000 customers and a churn rate of 1.5% per month. You invest in a Customer Success (CSM) team and a thorough program. In a year, your churn rate has come down to 1% per month. Was the investment worth it?
This is not a simple question to answer, because what if the CSM team is mostly saving low-revenue customers? What if they’re spending more in offers than they’ll be worth? Or what if they’re just buying 6 months of retention before the customer churns anyway?
Sales teams have long dealt with revenue projections, but those don’t always accurately take into account the future revenues from the portfolio of your existing customers. In order for the Chief Revenue Officer to do a comprehensive projection of their overall revenue, they must do a good job of estimating the LTV of their existing customers.
Most definitions of LTV are built around revenue. We think your use case should determine whether you use revenue or net revenue or a similar gain metric. For example, if you’re projecting company revenue as in the last use case above, it’s fine to look at estimated future revenues of your customers. But when considering what to spend on acquisition or retention, net revenue would be a better metric. For example, if you’re facing a churn risk and wondering how much to spend on the save attempt, you should not only consider that the customer pays you $500/month for your services, but also that it costs you $200/month to offer those services.
Going from a monthly gain from a customer to a lifetime gain from that customer requires you to estimate the remaining lifetime of the customer. One popular formula for that is 1/churn-rate which, of course, is an average rate of churn across all your customers. This link explains how to calculate the average churn rate and then how to use it to calculate the LTV estimate.
Formulas and rules-of-thumb might give you a good starting point but can rarely be used for meaningful analysis. For example, if I’m looking to exercise to lose weight, lots of good online sources will give me a formula for my target heart rate and the zone I should be in, e.g. (220-age)*60%to70%. A professional athlete, on the other hand, will employ a much more sophisticated science.
As a serious customer success athlete, you need to go beyond (1/churn-rate)*ARR as the way of assessing a customer’s value. First off, different customers have different churn tendencies and you cannot use the same churn rate across your entire customer base. Even if you divide your customer base into a few cohorts, this formula still doesn’t go very far. Not only would it ignore the differences between individual customers, but it also assumes that the churn rate and the ARR do not drastically change for the same customer over the lifetime of that customer.
A well-trained AI model can come to the rescue. With adequate amounts of data on your customers (more about that in a bit), such models can excel at:
Individual estimation:
Assuming we have different types of customer signals that SaaS companies today do a great job of maintaining, including product usage, support ticket data, detailed billing data, and customer feedback signals, we at StepFunction have had substantial success estimating the 2 key measures relevant here: (1) How much longer the customer will stay with you, and (2) What do you stand to gain from that customer over that period of time.
Cohort-level estimation:
Another benefit of well-crafted machine learning models is that they can improvise in cases of data deficiencies much better than formulas. If the customer has been with you only briefly, the model is able to intelligently match that customer to a set of peers and then build estimations from there.
In some cases, you’ll want to define the cohorts — for example, the small customers who signed up online in Q1 of 2021 for services costing less than $200/month.
There are other times when you don’t know how to define the cohorts and you want the machine to tell you. For example, the AI model might bucket together all self-registration customers who end up using 50% of the training and 50% of the features in the first month. As mentioned before, it does so as a way of improvising, or using proxy signals, in a world where holes in data are inevitable.
Having motivated the need for machine estimation of LTV, we’ve barely skimmed the surface on the implementation. We’ll address the requirements of adequate data in a subsequent post. The message I want to leave us with is that mature SaaS orgs with several years of customer data should think beyond simplistic formulas.
We helped our client adjust the incentives her team had put in place, and showed her beyond doubt how compelling the cost-to-benefit ratios were. It seemed like a lot of work to spend days preparing so many data signals and employing sophisticated machine learning models to do something that a formula could have done in minutes. But the difference in savings that this effort brought about and her confidence in those numbers made it all worth it. Another 10x ROI result from StepFunction.